Aims and scope
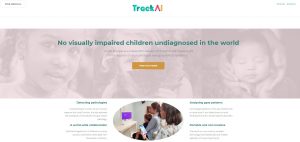
© Track AI website: https://dive-medical.com/TrackAI.html
The objective of the Track AI project (https://dive-medical.com/TrackAI.html) is to incorporate artificial intelligence (AI) into the DIVE medical device[1] so it can “estimate the probability of the patient having a certain pathology”. The innovation was created due to the difficulty in exploring the visual function (e.g. vision) among children, but it can be used for both collaborative and non-collaborative patients (i.e. patients who are able to collaborate with physicians as opposed to patients – such as children – who are unable to collaborate with physicians). The partnership allows technology to be shared between organisations and combines their experience in the health and technological fields.
The collaboration officially started in November 2018 and ended in December 2019. Three core partners were involved in the project: DIVE-Medical S.L. (the coordinator, a Spanish start-up that developed the DIVE Medical Device and which includes paediatric ophthalmologists from the Miguel Servet Hospital (Zaragoza, Spain) and engineers from the University of Zaragoza), Huawei (private partner, an international provider of ICT solutions) and IIS Aragón (public partner, health services research centre in Aragón). Some other organisations were also involved in the project, but with a lower level of participation: DIT Studio (a private software development company from Thailand) and five hospitals (public and private) from different countries (Spain, China, Mexico, Russia and Vietnam).
The innovation in this project consists of the development of a specific app that uses AI in a Huawei P30 mobile phone in order to analyse data from visual explorations using the DIVE-Medical device. This innovation helps physicians to determine whether or not a patient has a visual impairment by comparing patient gaze patterns with the database generated by previous diagnoses (which can be accessed online and offline). In some cases, it also makes it possible to estimate the probability of a particular pathology. The project is focused on non-collaborative patients, but it can also be used in collaborative patients (i.e. patients that are able to collaborate with physicians as opposed to patients – such as children – who are unable to collaborate with physicians).
Main collaborative innovation conditions
The initial ideas for Track AI emerged from the different knowledge and expertise (health and technological fields) of the partners and the interaction between partners that facilitated knowledge transfer. The actors looked for similarities between the ideas when there were different opinions (e.g. to find a specific diagnostic protocol), but there were not many disagreements because the objective and processes in the project were clearly defined from the very beginning.
The strategy followed was based on process agreements initiated by DIVE-Medical S.L.. Dive Medical S.L. and ISS Aragón were involved in concluding all agreements, the other partners were involved in those which affected them. Relationships between the partners and their participation in the project were defined in the contract and rules dealing with interactions between partners were established. Because of the highly contractual nature of the project, the public procurer can be considered to be the lead organization which governed the partnership, and took the crucial decisions (Provan and Kenis 2008). The shortage of specific knowledge about AI was overcome by including a new partner (DIT studio) that participated in developing part of the software.
The involvement of healthcare professionals was necessary because health knowledge and experience were crucial to design the tool and to define a user’s protocol for patients. Health care professionals were also needed for the test and piloting phases, specifically for patient selection, use of the innovation, and evaluation of the data obtained. Patient collaboration was needed for the test and piloting phases. The partners needed a large sample of patients from different countries to validate this tool. The failures detected by users were solved and their feedback and suggestions were recorded and implemented whenever possible (e.g. changes in the tests used and the classification of some parameters as normal or not).
Implications and lessons learned
The collaboration processes in this project have been smooth as the objectives and participation of all partners were perfectly defined in the contract. Conflicts in the innovation process were restricted to the differences in knowledge and experience between healthcare professionals and engineers. However, these were minor conflicts that have not affected the performance of the innovation process. Lack of knowledge in small parts of the innovation process was solved by subcontracting an external company. Focussing the project on just one objective in order to develop a specific innovation could have made it easier to align the goal with the efforts of the participants in this project.
The participation of users in this project was possible because of agreements with the hospitals that facilitated the access to enough patients. These hospitals mainly participated in the test and piloting phases and did not have any right over the developed technology. Their collaboration has been critical to obtain enough patients to validate the innovation in the future. Some difficulties arose during their participation, particularly due to data sharing issues because of differences in confidentiality laws that make it difficult to involve users from some countries.
The most important lessons learned in this project are the importance of defining an objective, flexibility to seek the knowledge needed in the partnership and communication with users. The definition of a clear objective and a specific innovation have contributed to making the innovation process easier and faster. The inclusion of another company when new knowledge was needed facilitated the design of the software. Continuous communication with users in the piloting phase of an innovation is essential to ensure the correct functioning of the new technology and take advantage of the feedback generated.
[1] Device for an Integral Visual Examination, a medical digital device for a fast and accurate exploration of the visual function of non-collaborative patients
To read more about the case study, see D7.1 – Practices of external collaboration for service delivery. Comparative case studies on external collaboration in eHealth partnerships report.
About the Authors
Lourdes Torres, University of Zaragoza
Vicente Pina, University of Zaragoza
Sonia Royo, University of Zaragoza
Jaime Garcia-Rayado, University of Zaragoza